公开数据集
数据结构 ?
6607.13M
Data Structure ?
* 以上分析是由系统提取分析形成的结果,具体实际数据为准。
README.md
## Overview
This dataset provides feature files extracted from *Tiny Imagenet Dataset*. These features are extracted through a [Python package](https://github.com/jgoodman8/py-image-features-extractor). These are the techniques used:
- Convolutional Neural Network Ensemble
- Linear Binary Patterns Histograms (LBPH)
- Bag of Features (bag-of-visual-words)
- SIFT
- SURF
- KAZE
## Ensemble
Notice that low-resolution images make harder to get feature vectors with good classification power. By applying *feature detection* methods, it is easy to check the number of detected *key-point*s. The volume of key-points is much elevated in higher resolution samples due to their better resolution. In fact, certain images do not present any key-point for the detectors applied. Consequently, the extracted features are likely to provide poor results.
In order to improve the quality of the extracted features, a new neural network has been built. The ConvNet architecture
is inspired by the stacking approach by combining extracted features with different fine-tuned networks. Thus, pre-trained
VGG19, ResNet50 and DenseNet201 are picked, fine-tuned over tiny epochs and their last layer is removed. As shown in
the figure, data is fed into these networks and their outputs are concatenated. Finally, two dense blocks and a softmax layer
are added at the top of the network. Each one of these dense blocks is built with a RELU layer, a batch normalization layer
and a dropout layer. The whole model is fine-tuned and the top blocks are removed to extract high-level features.
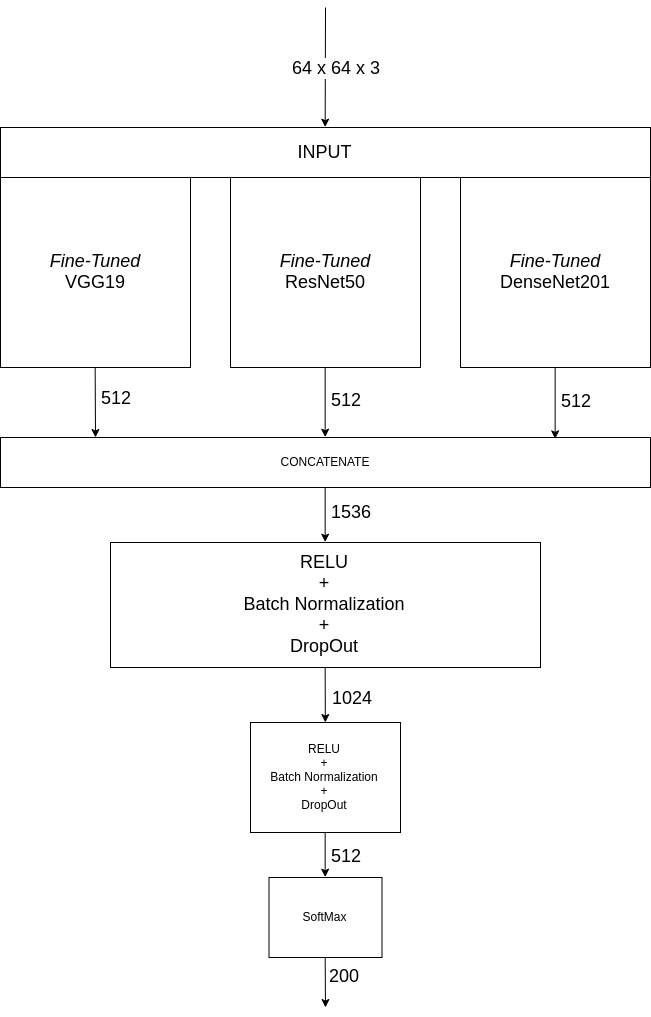
暂无相关内容。
暂无相关内容。
- 分享你的想法
去分享你的想法~~
全部内容
欢迎交流分享
开始分享您的观点和意见,和大家一起交流分享.
数据使用声明:
- 1、该数据来自于互联网数据采集或服务商的提供,本平台为用户提供数据集的展示与浏览。
- 2、本平台仅作为数据集的基本信息展示、包括但不限于图像、文本、视频、音频等文件类型。
- 3、数据集基本信息来自数据原地址或数据提供方提供的信息,如数据集描述中有描述差异,请以数据原地址或服务商原地址为准。
- 1、本站中的所有数据集的版权都归属于原数据发布者或数据提供方所有。
- 1、如您需要转载本站数据,请保留原数据地址及相关版权声明。
- 1、如本站中的部分数据涉及侵权展示,请及时联系本站,我们会安排进行数据下线。