公开数据集
数据结构 ?
547.98G
Data Structure ?
* 以上分析是由系统提取分析形成的结果,具体实际数据为准。
README.md
The nuScenes dataset is a large-scale autonomous driving dataset with 3d object annotations. It features:
¡ñ Full sensor suite (1x LIDAR, 5x RADAR, 6x camera, IMU, GPS)
¡ñ 1000 scenes of 20s each
¡ñ 1,400,000 camera images
¡ñ 390,000 lidar sweeps
¡ñ Two diverse cities: Boston and Singapore
¡ñ Left versus right hand traffic
¡ñ Detailed map information
¡ñ 1.4M 3D bounding boxes manually annotated for 23 object classes
¡ñ Attributes such as visibility, activity and pose
¡ñ New: 1.1B lidar points manually annotated for 32 classes
¡ñ New: Explore nuScenes on SiaSearch
¡ñ Free to use for non-commercial use
¡ñ For a commercial license contact nuScenes@motional.com
Data Collection
Scene planning
For the nuScenes dataset we collect approximately 15h of driving data in Boston and Singapore. For the full nuScenes dataset, we publish data from Boston Seaport and Singapore¡¯s One North, Queenstown and Holland Village districts. Driving routes are carefully chosen to capture challenging scenarios. We aim for a diverse set of locations, times and weather conditions. To balance the class frequency distribution, we include more scenes with rare classes (such as bicycles). Using these criteria, we manually select 1000 scenes of 20s duration each. These scenes are carefully annotated using human experts. The annotator instructions can be found in the devkit repository.
Car setup
We use two Renault Zoe cars with an identical sensor layout to drive in Boston and Singapore. The data was gathered from a research platform and is not indicative of the setup used in Motional products. Please refer to the above figure for the placement of the sensors. We release data from the following sensors:
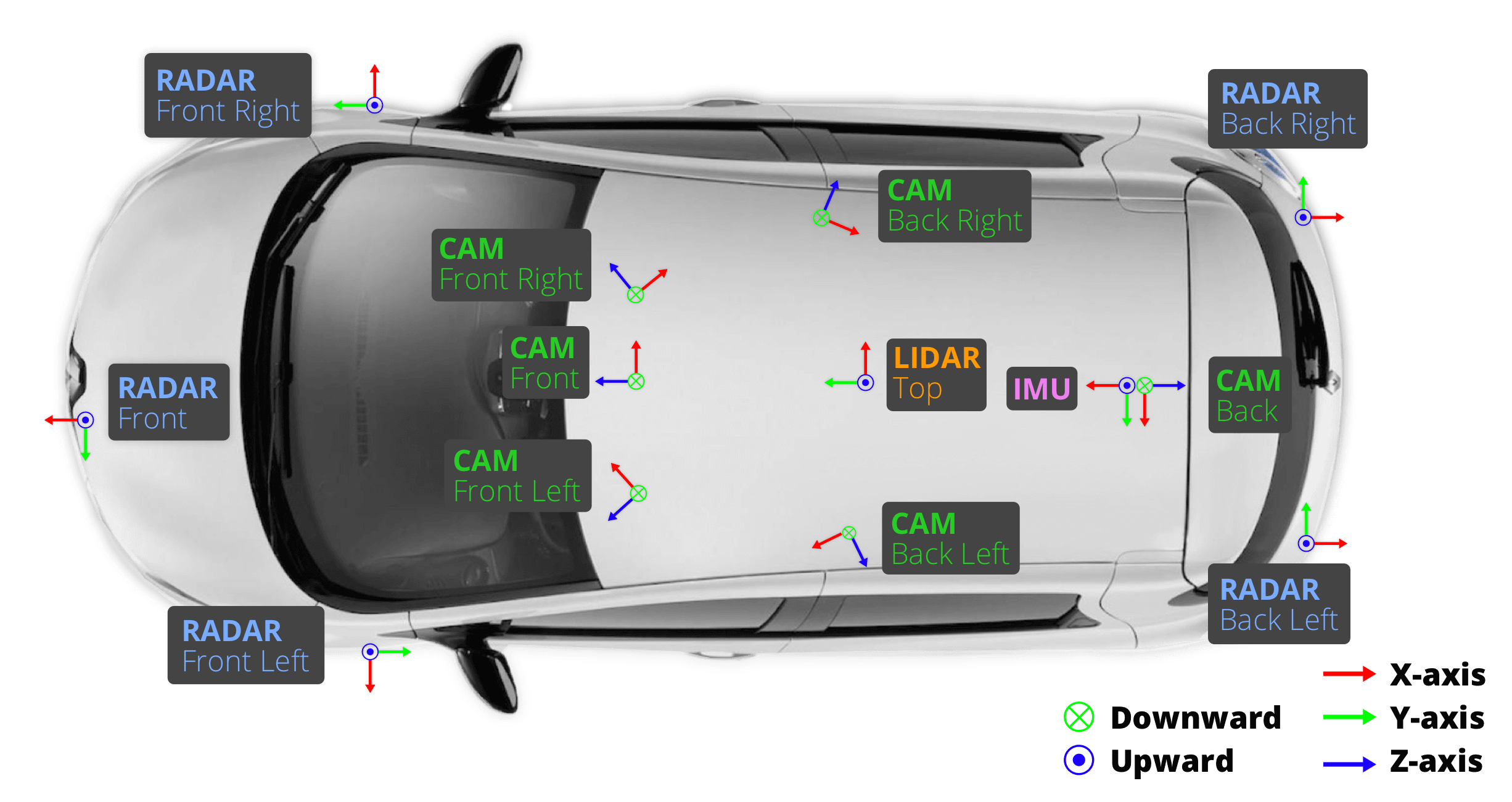
- 1x spinning LIDAR:
- 20Hz capture frequency
- 32 channels
- 360¡ã Horizontal FOV, +10¡ã to -30¡ã Vertical FOV
- 80m-100m Range, Usable returns up to 70 meters, ¡À 2 cm accuracy
- Up to ~1.39 Million Points per Second
- 5x long range RADAR sensor:
- 77GHz
- 13Hz capture frequency
- Independently measures distance and velocity in one cycle using Frequency Modulated Continuous Wave
- Up to 250m distance
- Velocity accuracy of ¡À0.1 km/h
- 6x camera:
- 12Hz capture frequency
- 1/1.8'' CMOS sensor of 1600x1200 resolution
- Bayer8 format for 1 byte per pixel encoding
- 1600x900 ROI is cropped from the original resolution to reduce processing and transmission bandwidth
- Auto exposure with exposure time limited to the maximum of 20 ms
- Images are unpacked to BGR format and compressed to JPEG
- See camera orientation and overlap in the figure below.
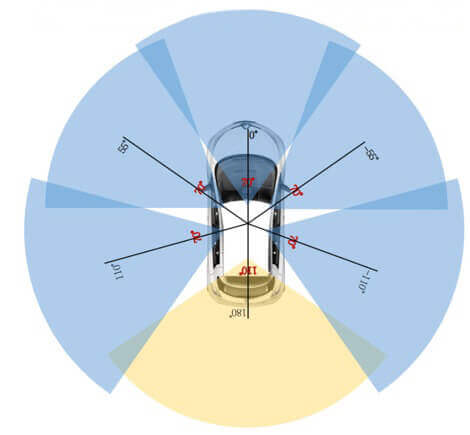
Sensor calibration
To achieve a high quality multi-sensor dataset, it is essential to calibrate the extrinsics and intrinsics of every sensor. We express extrinsic coordinates relative to the ego frame, i.e. the midpoint of the rear vehicle axle. The most relevant steps are described below:
-
LIDAR extrinsics:
We use a laser liner to accurately measure the relative location of the LIDAR to the ego frame.
-
Camera extrinsics:
We place a cube-shaped calibration target in front of the camera and LIDAR sensors. The calibration target consists of three orthogonal planes with known patterns. After detecting the patterns we compute the transformation matrix from camera to LIDAR by aligning the planes of the calibration target. Given the LIDAR to ego frame transformation computed above, we can then compute the camera to ego frame transformation and the resulting extrinsic parameters.
-
RADAR extrinsics
We mount the radar in a horizontal position. Then we collect radar measurements by driving in an urban environment. After filtering radar returns for moving objects, we calibrate the yaw angle using a brute force approach to minimize the compensated range rates for static objects.
-
Camera intrinsic calibration
We use a calibration target board with a known set of patterns to infer the intrinsic and distortion parameters of the camera.
Sensor synchronization
In order to achieve good cross-modality data alignment between the LIDAR and the cameras, the exposure of a camera is triggered when the top LIDAR sweeps across the center of the camera¡¯s FOV. The timestamp of the image is the exposure trigger time; and the timestamp of the LIDAR scan is the time when the full rotation of the current LIDAR frame is achieved. Given that the camera¡¯s exposure time is nearly instantaneous, this method generally yields good data alignment. Note that the cameras run at 12Hz while the LIDAR runs at 20Hz. The 12 camera exposures are spread as evenly as possible across the 20 LIDAR scans, so not all LIDAR scans have a corresponding camera frame. Reducing the frame rate of the cameras to 12Hz helps to reduce the compute, bandwidth and storage requirement of the perception system.
Privacy protection
It is our priority to protect the privacy of third parties. For this purpose we use state-of-the-art object detection techniques to detect license plates and faces. We aim for a high recall and remove false positives that do not overlap with the reprojections of the known person and car boxes. Eventually we use the output of the object detectors to blur faces and license plates in the images of nuScenes.
- 分享你的想法
全部内容
数据使用声明:
- 1、该数据来自于互联网数据采集或服务商的提供,本平台为用户提供数据集的展示与浏览。
- 2、本平台仅作为数据集的基本信息展示、包括但不限于图像、文本、视频、音频等文件类型。
- 3、数据集基本信息来自数据原地址或数据提供方提供的信息,如数据集描述中有描述差异,请以数据原地址或服务商原地址为准。
- 1、本站中的所有数据集的版权都归属于原数据发布者或数据提供方所有。
- 1、如您需要转载本站数据,请保留原数据地址及相关版权声明。
- 1、如本站中的部分数据涉及侵权展示,请及时联系本站,我们会安排进行数据下线。